Heidi Badgery, Managing Director of Alteryx ANZ, shares how we can build AI with integrity and neutralise biases.
In an era where data-driven insights increasingly deliver decision intelligence across science, government, and business sectors, the ability to unlock the full capacity of their ever-increasing data to make decisions faster is paramount. Data generation has reached unprecedented levels from social media and smart devices. Businesses must effectively leverage this data to extract genuine value in such a landscape. Amidst a challenging commercial landscape marked by macroeconomic pressures, forward-thinking leaders across various industry verticals are racing to take advantage of artificial intelligence and generative AI to improve productivity and accelerate time to insight.
Under constant pressure to guide their companies and help navigate volatile markets, it’s not merely about reacting to change but proactively anticipating it amidst constrained resources. With many gravitating towards the transformative potential of generative AI to reduce time to insights derived from data, 2024 is set to be the year the technology gains traction and moves to deliver business value. A recent Alteryx survey of 300 enterprise board members across four countries, including Australia, confirms that the sudden rise of generative AI has moved beyond hype and become a focus of the enterprise. Of the board members surveyed, 43% of Australians stated that generative AI is currently their “main priority above anything else”, and 39% are experimenting with generative AI in certain projects or departments.
Despite this interest in applying generative AI to tackle business challenges, the success of enterprise generative AI applications depends on employee adoption rates, use cases and willingness to use AI. However, they must also know the inherent risk of using imperfect data to train AI models or bypassing governed analytics processes developed by experts who understand the shape of data. So how can business leaders drive AI for all while ensuring the accuracy of the results produced by this technology?
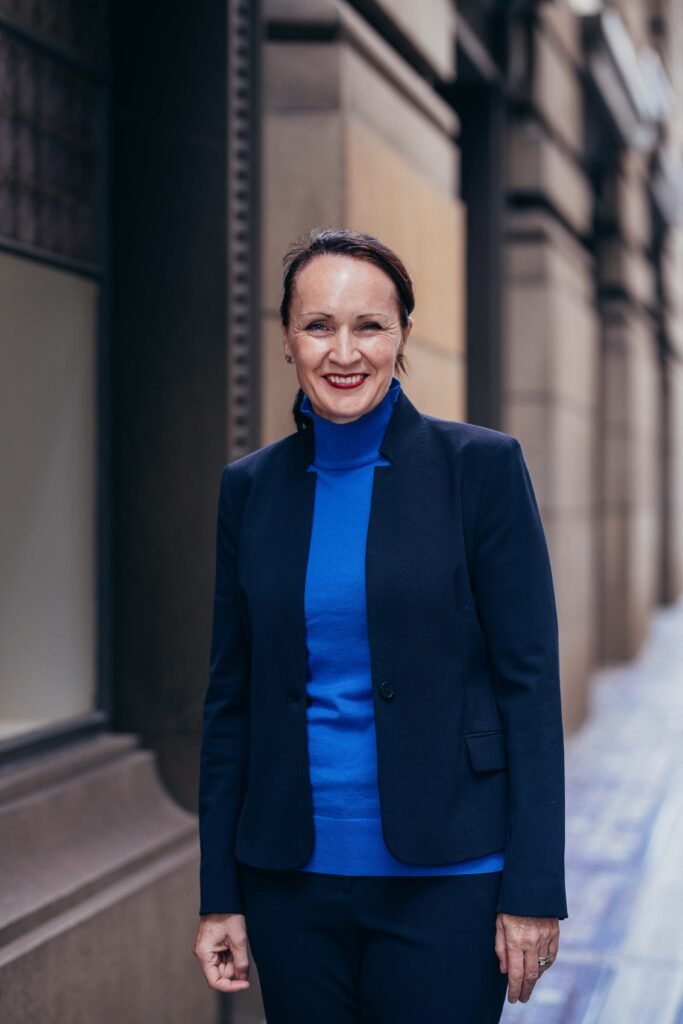
Challenges still to be met
As the use of artificial intelligence and generative AI becomes increasingly integrated into everyday life, concerns regarding access, accuracy, misinformation, bias, privacy, and security continue to stall mass adoption. This race to adoption has placed business leaders and governments under increasing pressure to understand and create sensible policy frameworks that determine how to create, maintain, and foster ethical and transparent AI. In fact, the Australian government recently launched an interim response on safe and responsible AI in Australia consultation to share their views on how to mitigate any potential risks of AI and support safe and responsible AI practices, including developing a voluntary AI Safety Standard, labelling and watermarking of AI-generated materials, and an expert advisory body.
Ultimately, it’s important to remember that any AI-driven system is only as good as the data it’s trained on and the ability of users to ask the right questions, implement the right data techniques, and understand the outcomes. Bad decisions can happen if people aren’t data and AI-literate. If you use it without knowing how to ask the right questions or without any knowledge around the data lineage used to train it, the outcomes will give you the wrong answers.
With bias and ethics remaining critical, AI solutions will only reflect our evolving ethical landscape if we institute mechanisms for recognising bias and incorporate the perspectives and experiences of a diverse spectrum of individuals right from the data collection phase. Addressing the issue of diversity in the development and model training phases is crucial, as rectifying it later will prove significantly more challenging.
Building AI with integrity
Just as buildings start with foundations, AI should begin with a firm base of data essentials – access, robust data governance frameworks, ETL, and analysis. Putting the best foot forward with these will result in accurate and ethical data pipelines of training data throughout the development lifecycle. As with any novel innovation, wise leaders must consider how technology might benefit their people and ultimately their business. Any AI-driven system is only as good as the data it’s trained on, but realising the value of AI across the enterprise requires employees who truly understand the data behind it.
In recent years, data used in models to automate the process of screening resumes have also been found to be biased against women applying for jobs and models used to assist judges in criminal sentence reviews have been found to be biased against black defendants – both had to be withdrawn as the data used needed careful human attention. The filling of a management position can also be similarly unfair if the information used for this purpose presents a very one-sided picture, as the legacy CVs used can be heavily originated from men, resulting in an inadvertent bias against women.
This situation highlights three essential criteria for ethical AI:
- Transparency and explainability—Understanding the data lineage of the pipeline used to train AI and how AI outcomes are produced is critical to assessing their integrity and accuracy.
- Human-in-the-loop oversight—Having robust oversight mechanisms which allow a “human-in-the-loop” or “human-in-command” approach to AI innovation is vital for ensuring that a human one that can hold the steering wheel on AI to audit and guide the AI’s operations while making use of its full capabilities.
- Data governance and privacy—Clear data governance frameworks prioritising end-to-end traceability, privacy, and security will be critical for guaranteeing models access to high-quality datasets created by governed analytics processes.
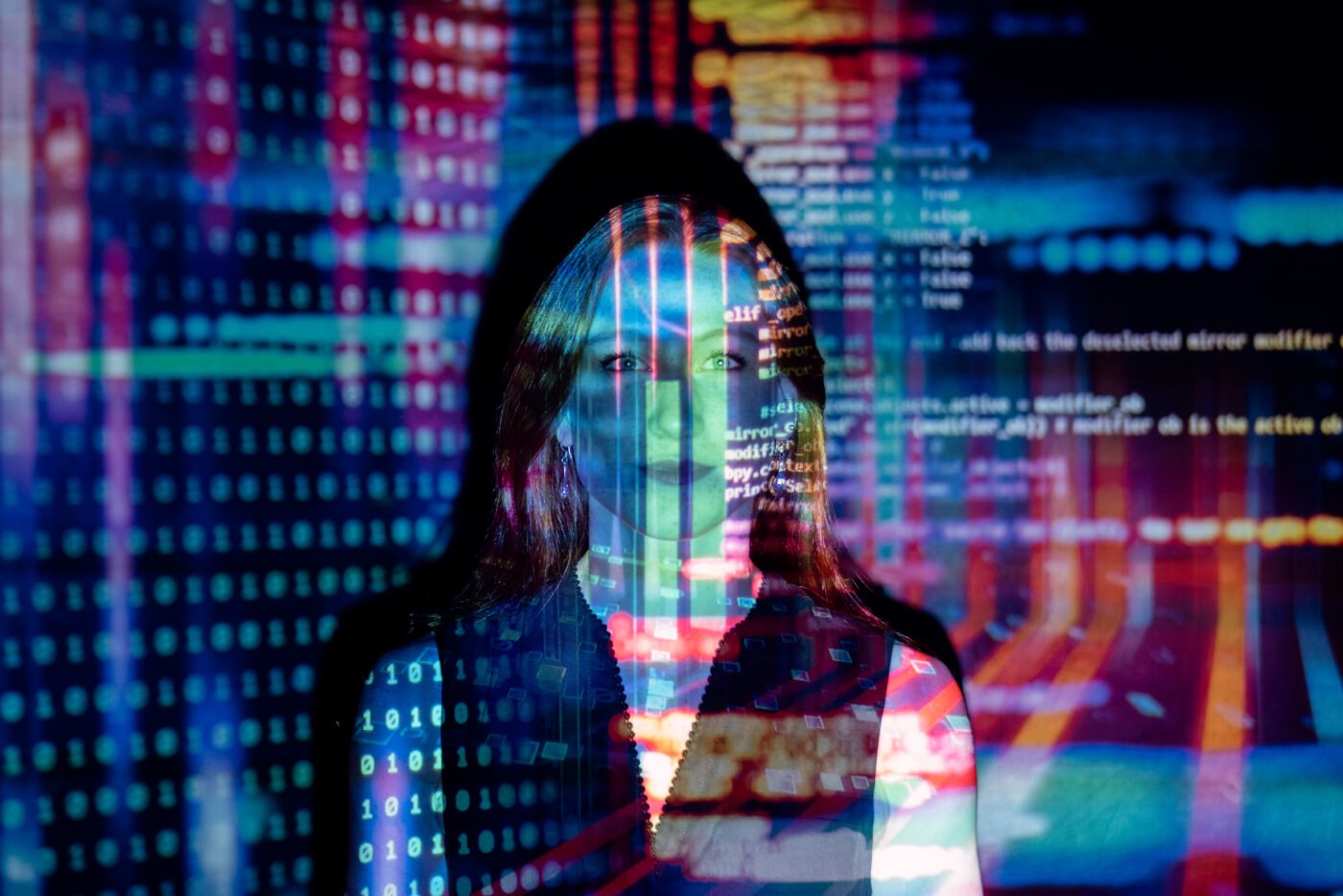
How can AI biases be neutralised?
Generative AI introduces new, intuitive and compelling ways for the business user – the accountant, the supply chain analyst, the merchandising analyst – to solve critical challenges with data by putting the power of better decision-making in everyone’s hands. Given how paramount quality data and data literacy are to delivering business value from generative AI, businesses may have difficulty fully understanding the appropriate use of the output without knowing what data went into training the model or how it works.
As with humans, AI keeps learning and improving. Ensuring it does so correctly require continual education and the development of strategies and policies that enshrine data privacy, ethics, and governance. Only by establishing and adopting best practices for identifying bias will businesses strengthen vigilance against potential problems while identifying and correcting inconsistencies from the outset.